Machine Learning: A First Course for Engineers and Scientists
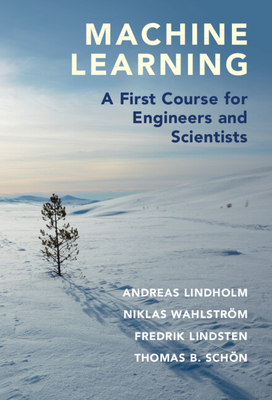
Machine Learning: A First Course for Engineers and Scientists
This book introduces machine learning for readers with some background in basic linear algebra, statistics, probability, and programming. In a coherent statistical framework it covers a selection of supervised machine learning methods, from the most fundamental (k-NN, decision trees, linear and logistic regression) to more advanced methods (deep neural networks, support vector machines, Gaussian processes, random forests and boosting), plus commonly-used unsupervised methods (generative modeling, k-means, PCA, autoencoders and generative adversarial networks). Careful explanations and pseudo-code are presented for all methods. The authors maintain a focus on the fundamentals by drawing connections between methods and discussing general concepts such as loss functions, maximum likelihood, the bias-variance decomposition, ensemble averaging, kernels and the Bayesian approach along with generally useful tools such as regularization, cross validation, evaluation metrics and optimization methods. The final chapters offer practical advice for solving real-world supervised machine learning problems and on ethical aspects of modern machine learning.
PRP: 614.75 Lei
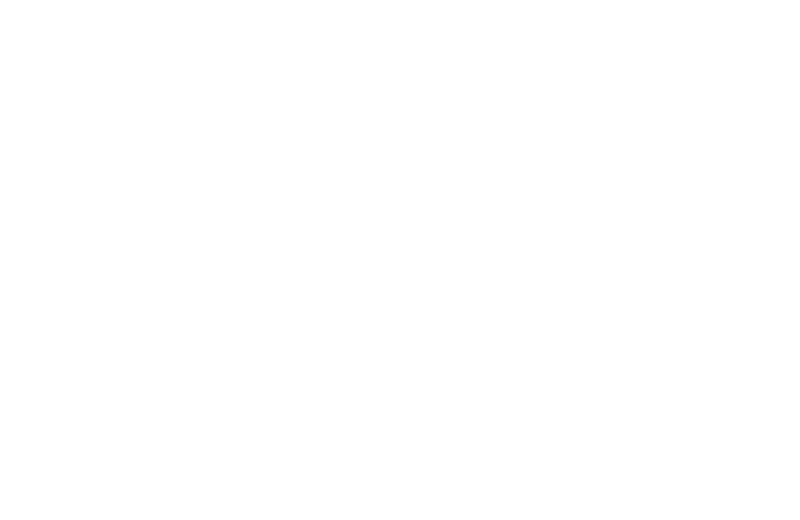
Acesta este Pretul Recomandat de Producator. Pretul de vanzare al produsului este afisat mai jos.
553.27Lei
553.27Lei
614.75 LeiLivrare in 2-4 saptamani
Descrierea produsului
This book introduces machine learning for readers with some background in basic linear algebra, statistics, probability, and programming. In a coherent statistical framework it covers a selection of supervised machine learning methods, from the most fundamental (k-NN, decision trees, linear and logistic regression) to more advanced methods (deep neural networks, support vector machines, Gaussian processes, random forests and boosting), plus commonly-used unsupervised methods (generative modeling, k-means, PCA, autoencoders and generative adversarial networks). Careful explanations and pseudo-code are presented for all methods. The authors maintain a focus on the fundamentals by drawing connections between methods and discussing general concepts such as loss functions, maximum likelihood, the bias-variance decomposition, ensemble averaging, kernels and the Bayesian approach along with generally useful tools such as regularization, cross validation, evaluation metrics and optimization methods. The final chapters offer practical advice for solving real-world supervised machine learning problems and on ethical aspects of modern machine learning.
Detaliile produsului