Neural Networks and Deep Learning: A Textbook
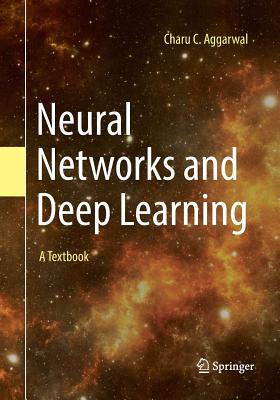
Neural Networks and Deep Learning: A Textbook
This book covers both classical and modern models in deep learning. The primary focus is on the theory and algorithms of deep learning. The theory and algorithms of neural networks are particularly important for understanding important concepts, so that one can understand the important design concepts of neural architectures in different applications. Why do neural networks work? When do they work better than off-the-shelf machine-learning models? When is depth useful? Why is training neural networks so hard? What are the pitfalls? The book is also rich in discussing different applications in order to give the practitioner a flavor of how neural architectures are designed for different types of problems. Applications associated with many different areas like recommender systems, machine translation, image captioning, image classification, reinforcement-learning based gaming, and text analytics are covered. The chapters of this book span three categories:
The basics of neural networks: Many traditional machine learning models can be understood as special cases of neural networks. An emphasis is placed in the first two chapters on understanding the relationship between traditional machine learning and neural networks. Support vector machines, linear/logistic regression, singular value decomposition, matrix factorization, and recommender systems are shown to be special cases of neural networks. These methods are studied together with recent feature engineering methods like word2vec.
Fundamentals of neural networks: A detailed discussion of training and regularization is provided in Chapters 3 and 4. Chapters 5 and 6 present radial-basis function (RBF) networks and restricted Boltzmann machines.
Advanced topics in neural networks: Chapters 7 and 8 discuss recurrent neural networks and convolutional neural networks. Several advanced topics like deep reinforcement learning, neural Turing machines, Kohonen self-organizing maps, and generative adversarial networks are introduced in Chapters 9 and 10.
The book is written for graduate students, researchers, and practitioners. Numerous exercises are available along with a solution manual to aid in classroom teaching. Where possible, an application-centric view is highlighted in order to provide an understanding of the practical uses of each class of techniques.
Descrierea produsului
This book covers both classical and modern models in deep learning. The primary focus is on the theory and algorithms of deep learning. The theory and algorithms of neural networks are particularly important for understanding important concepts, so that one can understand the important design concepts of neural architectures in different applications. Why do neural networks work? When do they work better than off-the-shelf machine-learning models? When is depth useful? Why is training neural networks so hard? What are the pitfalls? The book is also rich in discussing different applications in order to give the practitioner a flavor of how neural architectures are designed for different types of problems. Applications associated with many different areas like recommender systems, machine translation, image captioning, image classification, reinforcement-learning based gaming, and text analytics are covered. The chapters of this book span three categories:
The basics of neural networks: Many traditional machine learning models can be understood as special cases of neural networks. An emphasis is placed in the first two chapters on understanding the relationship between traditional machine learning and neural networks. Support vector machines, linear/logistic regression, singular value decomposition, matrix factorization, and recommender systems are shown to be special cases of neural networks. These methods are studied together with recent feature engineering methods like word2vec.
Fundamentals of neural networks: A detailed discussion of training and regularization is provided in Chapters 3 and 4. Chapters 5 and 6 present radial-basis function (RBF) networks and restricted Boltzmann machines.
Advanced topics in neural networks: Chapters 7 and 8 discuss recurrent neural networks and convolutional neural networks. Several advanced topics like deep reinforcement learning, neural Turing machines, Kohonen self-organizing maps, and generative adversarial networks are introduced in Chapters 9 and 10.
The book is written for graduate students, researchers, and practitioners. Numerous exercises are available along with a solution manual to aid in classroom teaching. Where possible, an application-centric view is highlighted in order to provide an understanding of the practical uses of each class of techniques.
Detaliile produsului