Practicing Trustworthy Machine Learning: Consistent, Transparent, and Fair AI Pipelines
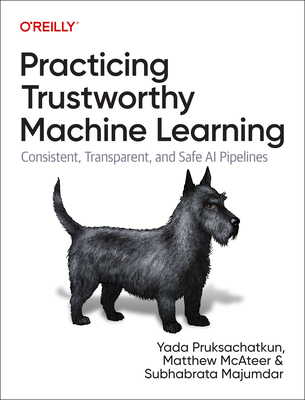
Practicing Trustworthy Machine Learning: Consistent, Transparent, and Fair AI Pipelines
With the increasing use of AI in high-stakes domains such as medicine, law, and defense, organizations spend a lot of time and money to make ML models trustworthy. Many books on the subject offer deep dives into theories and concepts. This guide provides a practical starting point to help development teams produce models that are secure, more robust, less biased, and more explainable.
Authors Yada Pruksachatkun, Matthew McAteer, and Subhabrata Majumdar translate best practices in the academic literature for curating datasets and building models into a blueprint for building industry-grade trusted ML systems. With this book, engineers and data scientists will gain a much-needed foundation for releasing trustworthy ML applications into a noisy, messy, and often hostile world.
You'll learn:
- Methods to explain ML models and their outputs to stakeholders
- How to recognize and fix fairness concerns and privacy leaks in an ML pipeline
- How to develop ML systems that are robust and secure against malicious attacks
- Important systemic considerations, like how to manage trust debt and which ML obstacles require human intervention
PRP: 495.94 Lei
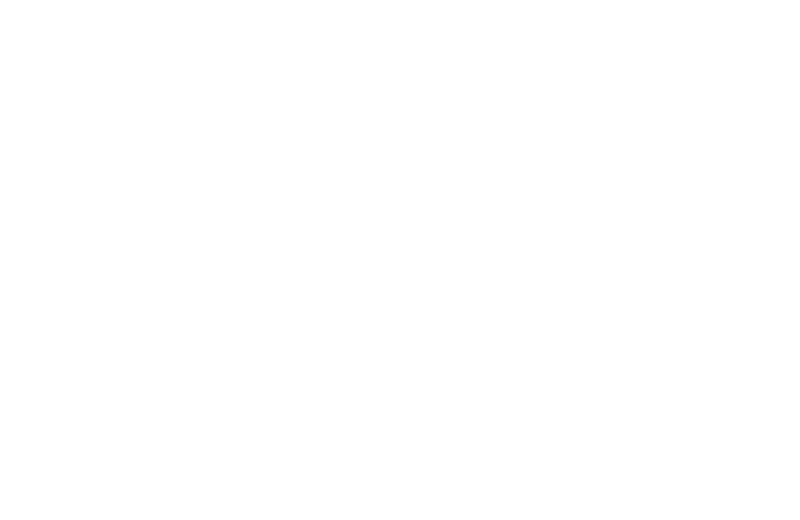
Acesta este Pretul Recomandat de Producator. Pretul de vanzare al produsului este afisat mai jos.
446.35Lei
446.35Lei
495.94 LeiLivrare in 2-4 saptamani
Descrierea produsului
With the increasing use of AI in high-stakes domains such as medicine, law, and defense, organizations spend a lot of time and money to make ML models trustworthy. Many books on the subject offer deep dives into theories and concepts. This guide provides a practical starting point to help development teams produce models that are secure, more robust, less biased, and more explainable.
Authors Yada Pruksachatkun, Matthew McAteer, and Subhabrata Majumdar translate best practices in the academic literature for curating datasets and building models into a blueprint for building industry-grade trusted ML systems. With this book, engineers and data scientists will gain a much-needed foundation for releasing trustworthy ML applications into a noisy, messy, and often hostile world.
You'll learn:
- Methods to explain ML models and their outputs to stakeholders
- How to recognize and fix fairness concerns and privacy leaks in an ML pipeline
- How to develop ML systems that are robust and secure against malicious attacks
- Important systemic considerations, like how to manage trust debt and which ML obstacles require human intervention
Detaliile produsului