Bayesian Hierarchical Models
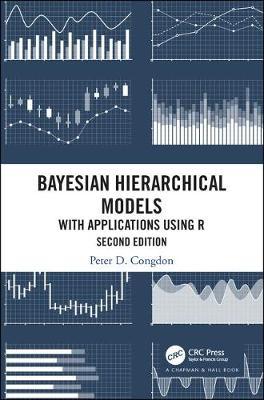
Bayesian Hierarchical Models
The new edition is a revision of the book Applied Bayesian Hierarchical Methods. It maintains a focus on applied modelling and data analysis, but now using entirely R-based Bayesian computing options. It has been updated with a new chapter on regression for causal effects, and one on computing options and strategies. This latter chapter is particularly important, due to recent advances in Bayesian computing and estimation, including the development of rjags and rstan. It also features updates throughout with new examples.
The examples exploit and illustrate the broader advantages of the R computing environment, while allowing readers to explore alternative likelihood assumptions, regression structures, and assumptions on prior densities.
Features:
Provides a comprehensive and accessible overview of applied Bayesian hierarchical modelling
Includes many real data examples to illustrate different modelling topics
R code (based on rjags, jagsUI, R2OpenBUGS, and rst
PRP: 906.49 Lei
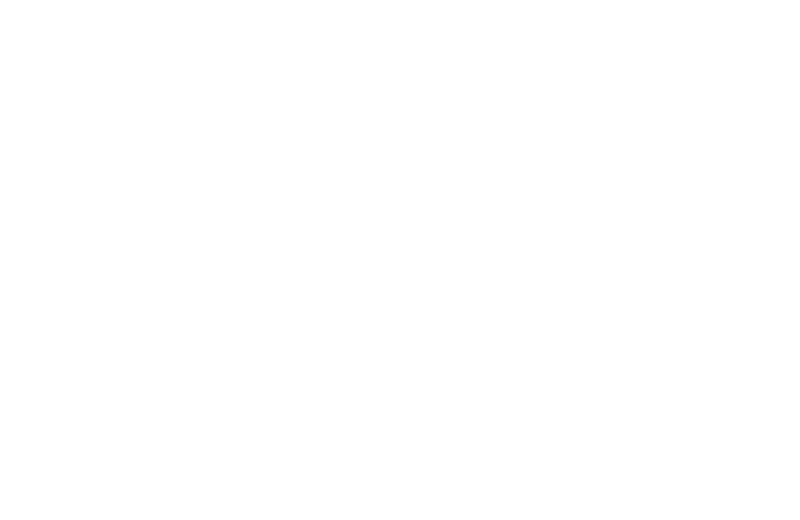
Acesta este Pretul Recomandat de Producator. Pretul de vanzare al produsului este afisat mai jos.
815.84Lei
815.84Lei
906.49 LeiLivrare in 2-4 saptamani
Descrierea produsului
The new edition is a revision of the book Applied Bayesian Hierarchical Methods. It maintains a focus on applied modelling and data analysis, but now using entirely R-based Bayesian computing options. It has been updated with a new chapter on regression for causal effects, and one on computing options and strategies. This latter chapter is particularly important, due to recent advances in Bayesian computing and estimation, including the development of rjags and rstan. It also features updates throughout with new examples.
The examples exploit and illustrate the broader advantages of the R computing environment, while allowing readers to explore alternative likelihood assumptions, regression structures, and assumptions on prior densities.
Features:
Provides a comprehensive and accessible overview of applied Bayesian hierarchical modelling
Includes many real data examples to illustrate different modelling topics
R code (based on rjags, jagsUI, R2OpenBUGS, and rst
Detaliile produsului